Food Innovation Quarterly: What inspired you to start the Emotion Research Lab?
Maria Pocovi: I started this project six years ago with the idea of connecting technology with humans. The key point is to make it possible for the technology to have the capacity, the ability, to read and to understand people’s emotions. The first step of our work has been to develop facial recording which means facial emotion recognition using computer vision and deep learning. So, we are an artificial intelligence company.
FIQ.: You work on facial emotion recognition and also on eye-tracking. About facial emotion recognition related to the food topic, I would like to understand how it works, how we can really understand the taste and the flavours.
M.P.: Regarding the food testing, at the beginning the companies started to use the technology for real analytics, to understand how people react in real time. One of our clients has been conducting research for product testing. He came to us and said: ‘Often it’s very difficult to really understand the emotions of the people. When they are testing new products, or when we need to move these products from different countries. It’s very difficult to see the real level of engagement with these new products’.
Taking emotions into account in purchasing strategies has become essential in a world where we are submerged by advertising messages that are sometimes contradictory: just think that the average attention span of a person to a stimulus today is only 5 seconds, whereas ten years ago it was 10 minutes!
So, we thought we can use our technology here. The testing experience starts from the first look at the product, and sometimes we also analyse the visual impact of the packaging. Traditionally, the results obtained through consumer surveys were most of the time biased, as much by the will, conscious or not, of the respondents not to tell the truth, by the way the questions are asked, by the influence of the environment in which the participants are placed, etc. By using neuroscientific techniques, such as facial recognition, we can learn the real insights of the consumer in real time.
I remember our first project in food; it was with an American company trying to bring a special yogurt to Mexico. In Mexico, as in many places, colour is connected with the flavour. In this new brand all of the yogurts had white colour, independent of the flavour. So you could have strawberry yogurt in white. People’s reactions were very strange about this. Some would say: ‘no, I don’t like the product’ whereas some really liked it, but overall, the emotion had been that of surprise, because they expected it to be pink!
FIQ.: Do you conduct research on the sensory experience itself (discovery of a new taste, an unknown flavour, a surprising texture)?
M.P.: Of course! It has been scientifically demonstrated that taste and smell are the senses that send the most information to the brain and generate the most memories in the eater. The algorithms we have set up transform these reactions into primary and secondary emotions. This ‘translation’ allows us to detect both pleasure and aversion for certain foods, as well as the emotional activation generated by the experience of different flavours and textures.
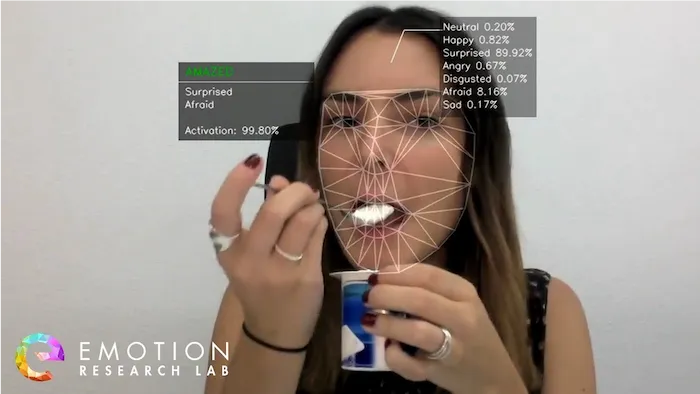
FIQ.: I guess the ways we react in Europe are not really different between Spain, France, England or Belgium… But it would be a much bigger difference if we have to make comparisons with countries from Africa, or Asia. How is the machine learning able to understand this difference?
M.P.: The key point is the dataset. You need to work with huge datasets, and they must be balanced. They must include all the ethnicities. Machine learning models read the different elements when people are expressing different emotions. When you are working with deep learning technology, every project starts with building a dataset. Artificial intelligence would be the perfect tool but, currently, there is still a human element for levelling images, videos…
You can work with different ethnicity, but in any case, the level of accuracy is subject to having a balanced dataset with a big number of images that really allows to work in global environments. With facial coding, the problem is that sometimes datasets are only trained for one ethnicity. So, depending on the project, it’s best to use the appropriate model. If you work on a Caucasian subject with Asian dataset, the result would be a disaster (laugh).
FIQ.: The way we express ourselves is also a question of cultural values. Are these also included in your project and in the datasets?
M.P.: No, datasets are only working with facial expressions. I understand your point and, of course, human emotions are a very complex challenge. So, when we talk about how humans express emotions, you obviously need to consider the voice, the face, the movements, but first of all you really need to consider the context. At this point, we are talking about facial coding and having information in a scalable way. You should also consider that earlier, clients were analysing the videos manually.
Let’s not forget that deep learning is a recent field of research. The amount of data we collect is growing, as are the analytical models. At Emotion Research LAB, we fully assume that our work is constantly evolving!
FIQ.: About facial emotional recognition, there are a lot of aspects: emotion detection, emotional metrics, moods, secondary emotions… What do you call a secondary emotion… How do you distinguish between these different emotional levels?
M.P.: Our work is based on the work of American psychologists such as Paul Ekman or Robert Plutchik, particularly on his theory of the ‘wheel of emotions’. According to him, our emotional system is structured around six basic sensations (happiness, surprise, anger, disgust, fear and sadness) from which an infinite number of more subtle emotions unfold. These secondary emotions are essential because they allow us to understand in detail the behaviour and feelings of an individual or a group of individuals. Reading a secondary emotion comes from the basic emotions, but the algorithm has to connect a combination of the basic emotion with their intensity.
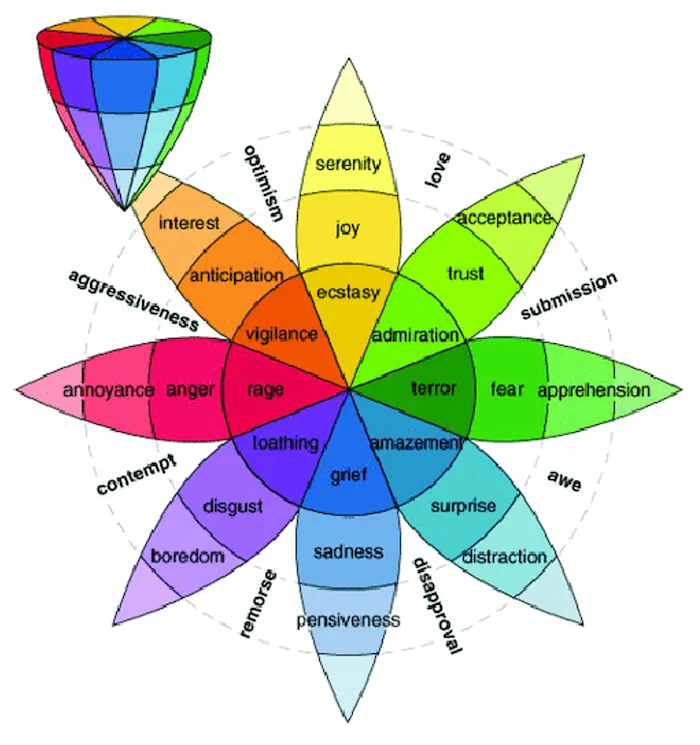
However, in practice, the treatment of these secondary emotions often presents us with difficulties of interpretation: surprise can be the manifestation of a simple intake of information or, on the contrary, of irritation, just as happiness can at the same time be manifested by pleasure, optimism or ecstasy. The division between primary sensations and secondary emotions is therefore only theoretical: our algorithms are precisely parameterized to analyze their combination and their respective intensity.
FIQ. : Is facial emotional recognition also combined with eye tracking?
M.P. : We actually use guesstimation and not eye tracking. Eye tracking uses infrared solutions and is more accurate. We are trying to reduce the amount of uncertainty which means constantly developing new analysis tools. Eye tracking is one of them and it is a good complement to our facial recognition models.
FIQ. : What market sectors does the Emotion Research Lab operate in today?
M.P. : Our clients are very global. For example, we work with companies in the USA, to understand experiences with real time applications. So, you have cameras in a store, and you can analyse people’s reactions while they are shopping. We work with a company in Dubai and Mexico, for their webspace innovation. We worked with a company in China to introduce this emotion recognition in smart sales process. Of course, we also work with companies for food testing, with big companies, when they have to launch new products, like ice cream (laughs).
FIQ. : In the coming future, what would be more important? Real life analytics or the usual testing?
M.P. : I don’t know, because the Covid situation has changed the game. Online activity is becoming very necessary while face to face is going to be more complicated. In real analytics, it’s going to be a combination. For example, we are now launching one application with a new deep learning model to understand if people are wearing a mask or not, for safety reasons. Computer based things are always a challenge for us, we enjoy to do that.
The other day, I was talking to a good client in the USA who prefers to close the office and maintain remote working because it would be complicated if something happens to his employees. At the moment everything still must be done online. We need to see how the market is going to react during 2021 but, the sure thing is that the new clients and their demands are centred around online solutions.
FIQ. : When someone creates a machine, he or she is usually the very first one to try it in order to challenge its efficiency, right? Were you the first ever person to use your application, and did you video record yourself to test the analysis program?
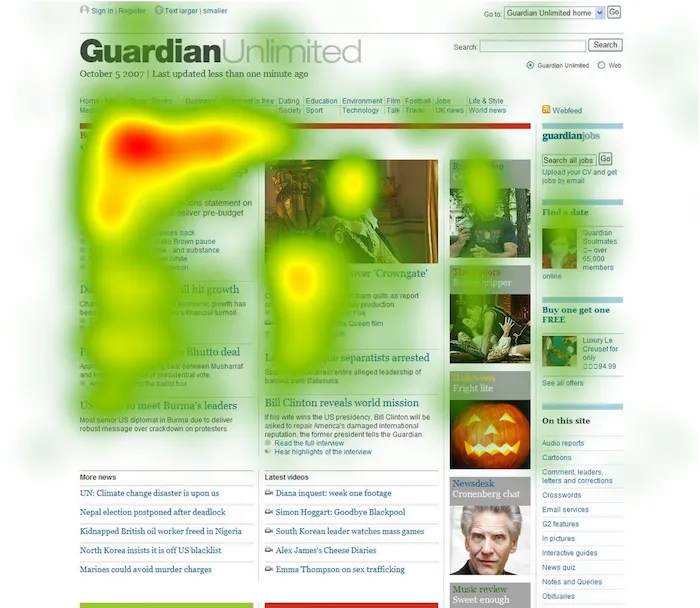
M.P. : Myself? (laughs), Yes, I have done it, of course… I believe first it was Alicia, the co-founder of the company, she’s on the technological side. So, she was the first person to do it and, after that, I did this test on myself.
FIQ. : Did you find it disturbing? Not especially the real time thing, but more about how it really works? I think it must be a bit different when the tester is yourself, is not it?
M.P. : A bit. You put yourself in front of the camera and then read your emotional reactions. You can’t control your emotions because one of the points of the technology is that you can’t lie to the machine. But I am not the right person because, in the end, I know the technology… For example, I know the angles from where the machine can not the read my face (laughs)
FIQ. : (laughs) So did you cheat?
M.P. : (laughs) For instance, many times, companies or clients ask us in the exhibitions to allow the people to see their own emotional reactions and it’s hilarious.
Of course, the first time, people are a bit surprised, but eventually they enjoy it so much, staying in front of the screen looking at their own emotions, they talk, they feel and see emotions, they act like kids.
FIQ. : It’s not really surprising when you know that most of the people already like to take selfies, this surely sounds like a game to them.
M.P. : Yes! I believe the culture is changing in the way that people are now increasingly into visual, they like to see everything but, most importantly, they want to show their own emotions.
FIQ. : Emotion Research Lab is not the only company in the ‘digital emotion market’? Affectiva also works in the same field… What would be the big differences between the two companies?
M.P. : She, because CEO and founder is also a woman, has been in this business since the beginning. Rana el Kaliouby started Affectivas as a spin off from MIT, so they have the investment from it, and a very powerful network. Now they are focused on the future of the automotive, and how emotions can be connected. Because it’s from the MIT, the only thing that I can tell about them is that I’m very proud to be in the market that people consider us as competitor.
We have been focused on how we can explain the emotional reaction deeper and to understand the secondary emotions. And I believe for companies like us, the challenge is the context. This is why we are now including masks;
this is why we are working on objects identification, and I believe the way that you can improve the best is to make ‘understanding the context’ possible.
Our approach is more visual and contextual while they are working with voice recognition. So, I believe we are taking the solution from a different angle. In the number of emotions that we can create, we are reading more emotions than Affectiva does, because the approach is different.
FIQ. : How do you imagine yourself and your company in 10 years?
M.P. : (laughs) No… Seriously, in 10 years, for me, the answer is obvious: a better consideration of the geocultural context in which our emotions are expressed. Our roadmap is clear, even if it may seem ambitious: the next ten years must allow us to build models for the analysis of emotional experiences that do not simply take into account facial expressions or non-verbal communication elements, but also all cultural norms and habitus.
Interviewed by Cyprien Rose for Food Innovation Quarterly